ES7 嵌套分桶的过滤与排序
工作中遇到一个复杂的需求:先根据IP字段为Syslog日志分组,再在每个组中按日志类型(思科路由器、华为防火墙、Jinper防火墙等)分组,但是却没有日志类型的字段,并且要实现多字段排序,另外 Java端还要根据IP、日志类型过滤。下面直接贴参考语法。
第1步:首先来解决嵌套分组
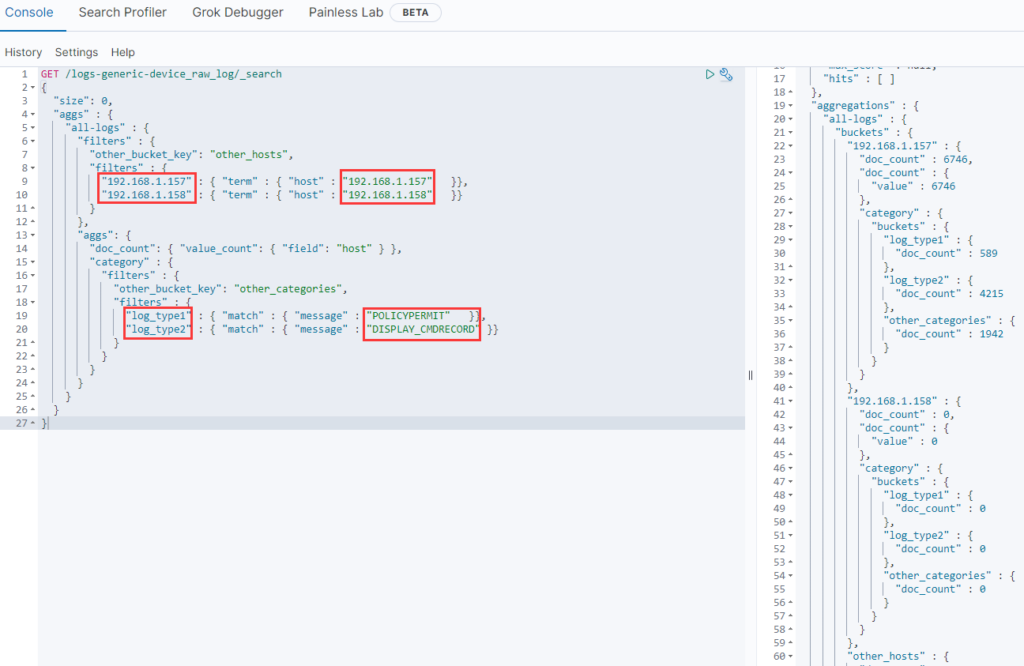
上面的查询语句按IP分了三个桶,分别是 192.168.1.157、192.168.1.158 和 other_hosts,接着在每个IP桶里又嵌套了3个日志类型的桶,分别是 log_type1、log_type2、other_categories。
圈出来的地方是要根据客户在页面上的选择进行过滤的,需要在 Java 查询代码里动态设置。
由于日志类型的桶在 ElasticSearch 数据库中是没有字段的,因此这里使用了 match 匹配,匹配到某一类的都归那类日志。
第2步:嵌套统计与多字段排序
有了上面的知识,我们再来看实际的代码,无非加了统计数量和排序:
请求
{ "size": 0, "aggs" : { "a1" : { "filters" : { "other_bucket_key": "other_hosts", "filters" : { "192_168_1_157" : { "term" : { "host" : "192.168.1.247" }}, "192_168_1_247" : { "term" : { "host" : "192.168.1.249" }} } }, "aggs": { "doc_count2": { "value_count": { "field": "host" } }, "a2" : { "filters" : { "other_bucket_key": "other_categories", "filters" : { "log_type1" : { "match_phrase" : { "message" : "type=conn" }}, "log_type2" : { "match_phrase" : { "message" : "type pf" }}, "log_type3" : { "bool": { "should": [ { "match_phrase": { "message": "type pf" }}, { "match_phrase": { "message": "type=conn" }} ] } } } }, "aggs": { "doc_count3": { "value_count": { "field": "host" } }, "sales_bucket_sort2": { "bucket_sort": { "sort": [ { "doc_count3": { "order": "asc" } } ] } } } }, "sales_bucket_sort": { "bucket_sort": { "sort": [ { "doc_count2": { "order": "asc" } } ] } } } } } }
结果
{ "took" : 523, "timed_out" : false, "_shards" : { "total" : 1, "successful" : 1, "skipped" : 0, "failed" : 0 }, "hits" : { "total" : { "value" : 2239, "relation" : "eq" }, "max_score" : null, "hits" : [ ] }, "aggregations" : { "a1" : { "buckets" : { "192_168_1_157" : { "doc_count" : 4, "a2" : { "buckets" : { "other_categories" : { "doc_count" : 4, "doc_count3" : { "value" : 4 } } } }, "doc_count2" : { "value" : 4 } }, "other_hosts" : { "doc_count" : 8, "a2" : { "buckets" : { "other_categories" : { "doc_count" : 8, "doc_count3" : { "value" : 8 } } } }, "doc_count2" : { "value" : 8 } }, "192_168_1_247" : { "doc_count" : 2227, "a2" : { "buckets" : { "log_type1" : { "doc_count" : 33, "doc_count3" : { "value" : 33 } }, "other_categories" : { "doc_count" : 126, "doc_count3" : { "value" : 126 } }, "log_type2" : { "doc_count" : 2068, "doc_count3" : { "value" : 2068 } }, "log_type3" : { "doc_count" : 2101, "doc_count3" : { "value" : 2101 } } } }, "doc_count2" : { "value" : 2227 } } } } } }
上面的 doc_count2 存储了每个IP桶里有多少条数据, doc_count3 则存储了每个IP桶里的每个日志类型桶里有多少条数据。 另外我在 doc_count2 上进行了 asc 排序,在 doc_count3 也进行了 asc 排序,这和关系数据库里的多字段排序是一个意思,先按 doc_count2 排,再按 doc_count3 排。
FiltersAggregationBuilder 过滤聚合类
上面嵌套分组聚合例子中,原始数据中是没有分组信息的,而是过滤出来的分组信息。在 Java 中这个过滤聚合类对应的是 FiltersAggregationBuilder
。
再来看个查询:类型 t-shirt 的平均价格,先根据类型过滤,然后在进行聚合计算。
{ "aggs" : { "t_shirts" : { "filter" : { "term": { "type": "t-shirt" } }, "aggs" : { "avg_price" : { "avg" : { "field" : "price" } } } } } }
Java Elasticsearch 聚合查询(Aggregation)详解
ElasticSearch Java 客户端与聚合
先按ruleUuid 值构建 buckets,再在 bucket 里嵌套 srcIP 值的 bucket:
GET /_search { "size": 0, "aggs": { "ruleUuidBuckets": { "terms": { "field": "ruleUuid" }, "aggs": { "srcIPBuckets": { "terms": { "field": "srcIP" } } } } } }
如果要对 ruleUuid、srcIP 进行 distinct 操作,那么上面的聚合方式就够了,结果:
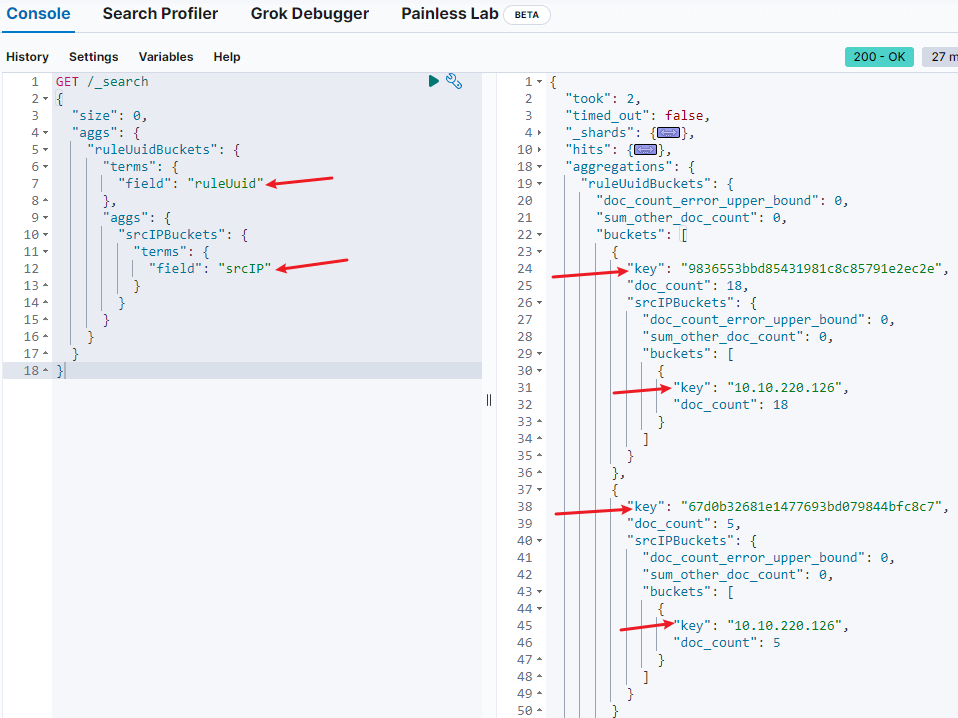
对应的 elasticsearch java 实现:
public Map<String, FilterRuleAggregation> distinctSrcIPGroupByFilterRuleUuid(Collection<String> ruleUuids) { Map<String, FilterRuleAggregation> ruleAggMap = new LinkedHashMap<>(); List<FieldValue> fieldValues = ruleUuids.stream().map(FieldValue::of).collect(Collectors.toList()); TermsQueryField termsQueryField = TermsQueryField.of(t -> t.value(fieldValues)); Query byRuleUuids = QueryBuilders.terms(t -> t.field("ruleUuid").terms(termsQueryField)); SearchRequest searchRequest = SearchRequest.of(r -> r .index(ESPolicyLog.INDEX_NAME) .size(0) //无需返回 resp.hits() 值 .query(byRuleUuids) .aggregations("ruleUuidBuckets", b -> b .terms(t -> t.field("ruleUuid")) .aggregations("srcIPBuckets", s -> s .terms(t -> t.field("srcIP"))))); try { SearchResponse<Object> resp = esClient.search(searchRequest, Object.class); List<StringTermsBucket> ruleUuidBuckets = resp.aggregations().get("ruleUuidBuckets") .sterms().buckets().array(); for (StringTermsBucket ruleUuidBucket : ruleUuidBuckets) { String ruleUuid = ruleUuidBucket.key().stringValue(); FilterRuleAggregation ruleAgg = new FilterRuleAggregation(ruleUuid); List<StringTermsBucket> srcIPBuckets = ruleUuidBucket.aggregations().get("srcIPBuckets") .sterms().buckets().array(); for (StringTermsBucket srcIPBucket : srcIPBuckets) { String srcIP = srcIPBucket.key().stringValue(); long docCount = srcIPBucket.docCount(); ruleAgg.add(new FilterRuleSrcIPBucket(srcIP, docCount)); } if (CollectionUtils.isNotEmpty(ruleAgg.getSrcIPBuckets())) { ruleAggMap.put(ruleUuid, ruleAgg); } } return ruleAggMap; } catch (Throwable ex) { throw new RuntimeException(ex); } }
小技巧:searchRequest.toString() 可生成 kibana 的 dev_tools 里测试的API json 参数,方便理解 Java 客户端得的使用。
ES8 嵌套分桶的过滤与排序
由于要存储时序数据,被迫升级到 ES8,下面使用 ElasticSearch Java 客户端来改造。
Java端代码:
// 先按 host 分组,再嵌套 category 分组(这里的 host 不是个字段,它是 keyed,汇聚后由 filters生成) @Override public List<DeviceRawLogSubtotal> groupByHostAndTypeDesc(String host, String vsysName, String syslogMatchIP, String message, Date startDatetime, Date endDatetime, Collection<String> excludeIps, List<String> agg1Hosts, Map<String, List<String>> agg2Categories) { BoolQuery.Builder boolQuery = getQuery(host, vsysName, syslogMatchIP, message, startDatetime, endDatetime, excludeIps); Map<String, Query> hostkeyedQueryMap = new LinkedHashMap<>(); for (String hostStr : agg1Hosts) { Query keyedQuery = QueryBuilders.term(t -> t.field("host").value(hostStr)); hostkeyedQueryMap.put(hostStr, keyedQuery); } FiltersAggregation hostFiltersAgg = new FiltersAggregation.Builder() .filters(c -> c.keyed(hostkeyedQueryMap)) .otherBucketKey("other_host") .build(); Map<String, Query> categoryKeyedQueryMap = new LinkedHashMap<>(); for (Map.Entry<String, List<String>> entry : agg2Categories.entrySet()) { String logType = entry.getKey(); List<String> patterns = entry.getValue(); for (String pattern : patterns) { Query keyedQuery = QueryBuilders.matchPhrase(t -> t.field("message").query(pattern)); categoryKeyedQueryMap.put(logType, keyedQuery); } } FiltersAggregation categorySubFiltersAgg = new FiltersAggregation.Builder() .filters(c -> c.keyed(categoryKeyedQueryMap)) .otherBucketKey("other_category") .build(); // BucketSortAggregation hostSortAgg=BucketSortAggregation SortOptions hostAggSortOptions = SortOptions.of(s -> s .field(FieldSort.of(f -> f .field("doc_count") .order(SortOrder.Desc)) ) ); SearchRequest searchRequest = SearchRequest.of(r -> r .index(DeviceRawLog.INDEX_NAME) .size(0) //无需返回 resp.hits() 值 .query(boolQuery.build()._toQuery()) .aggregations("hostBuckets", h -> h .filters(hostFiltersAgg) .aggregations("doc_count", d -> d.valueCount(c -> c.field("host"))) .aggregations("hostBucketSort", c -> c.bucketSort(b -> b.sort(hostAggSortOptions))) .aggregations("categoryBuckets", c -> c .filters(categorySubFiltersAgg) .aggregations("doc_count", d -> d.valueCount(e -> e.field("host"))) .aggregations("hostDocCountSort", d -> d.bucketSort(b -> b.sort(hostAggSortOptions))) ) ) ); String apiRequest = searchRequest.toString(); try { List<DeviceRawLogSubtotal> result = new ArrayList<>(); SearchResponse<Object> resp = esClient.search(searchRequest, Object.class); Buckets<FiltersBucket> filtersBucketBuckets = resp.aggregations().get("hostBuckets").filters().buckets(); // kibana测试返回的数据是经过排序的,但存储到 hostBucketMap 后却无顺了 Map<String, FiltersBucket> hostBucketMap = filtersBucketBuckets.keyed(); List<DeviceRawLogSubtotal.LogTypeSubtotal> logTypeSubtotals = new ArrayList<>(); for (Map.Entry<String, FiltersBucket> entry : hostBucketMap.entrySet()) { String keyHost = entry.getKey(); FiltersBucket bucket = entry.getValue(); DeviceRawLogSubtotal subtotal = new DeviceRawLogSubtotal(keyHost, bucket.docCount(), logTypeSubtotals); result.add(subtotal); Aggregate categoryAggregate = bucket.aggregations().get("categoryBuckets"); Buckets<FiltersBucket> catFiltersBucketBuckets = categoryAggregate.filters().buckets(); // kibana测试返回的数据是经过排序的,但存储到 catBucketMap 后却无顺了 Map<String, FiltersBucket> catBucketMap = catFiltersBucketBuckets.keyed(); for (Map.Entry<String, FiltersBucket> subEntry : catBucketMap.entrySet()) { String category = subEntry.getKey(); FiltersBucket catBucket = subEntry.getValue(); DeviceRawLogSubtotal.LogTypeSubtotal logTypeSubtotal = new DeviceRawLogSubtotal.LogTypeSubtotal( category, catBucket.docCount()); logTypeSubtotals.add(logTypeSubtotal); } } // ES8直接排序虽起作用,但Java端接收用 Map 类型导致排序被打乱,这里使用 stream.sort() 修复 result = result.stream().sorted(Comparator.comparing(DeviceRawLogSubtotal::getCount)).collect(Collectors.toList()); for (DeviceRawLogSubtotal item : result) { if (item.getLogTypeSubtotal() == null) continue; item.setLogTypeSubtotal(item.getLogTypeSubtotal().stream().sorted(Comparator.comparing(DeviceRawLogSubtotal.LogTypeSubtotal::getCount).reversed()).collect(Collectors.toList())); } return result; } catch (Throwable ex) { throw new RuntimeException(ex); } }
将生成的 api 请求放 kibana中,检查返回结果:
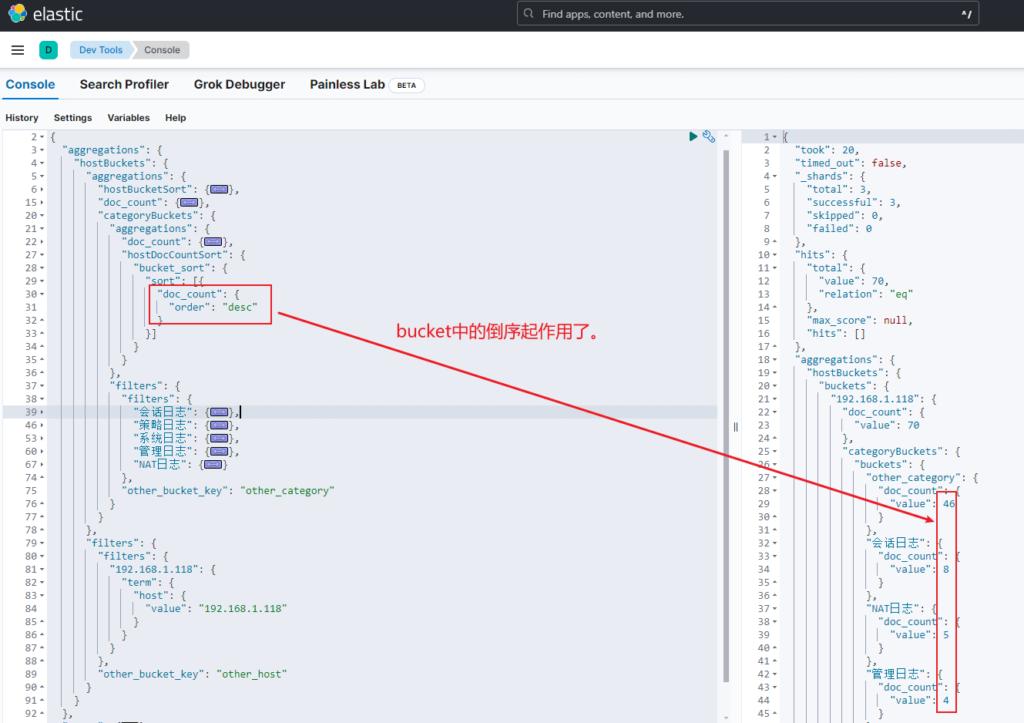
虽然上面排序没有问题,但是java客户端接收时却用的 Map,导致排序被打乱了,这是不是一个BUG?还是我用错方法了?
生成的完整 api 请求参数:
POST /logs-generic-device_raw_log/_search { "aggregations": { "hostBuckets": { "aggregations": { "hostBucketSort": { "bucket_sort": { "sort": [{ "doc_count": { "order": "desc" } }] } }, "doc_count": { "value_count": { "field": "host" } }, "categoryBuckets": { "aggregations": { "doc_count": { "value_count": { "field": "host" } }, "hostDocCountSort": { "bucket_sort": { "sort": [{ "doc_count": { "order": "desc" } }] } } }, "filters": { "filters": { "会话日志": { "match_phrase": { "message": { "query": "type=conn" } } }, "策略日志": { "match_phrase": { "message": { "query": "type=pf" } } }, "系统日志": { "match_phrase": { "message": { "query": "type=system" } } }, "管理日志": { "match_phrase": { "message": { "query": "type=mgmt" } } }, "NAT日志": { "match_phrase": { "message": { "query": "type=ac" } } } }, "other_bucket_key": "other_category" } } }, "filters": { "filters": { "192.168.1.118": { "term": { "host": { "value": "192.168.1.118" } } } }, "other_bucket_key": "other_host" } } }, "query": { "bool": { "must": [{ "bool": {} }] } }, "size": 0 }
注意!aggs 聚合结果中默认最多返回10个 buckets
策略利用率功能发布到客户环境后计算结果总是不对,将容器 data 数据拿回来测试,发现aggs总是返回10个,经查此文,应该默认最多返回10个,解决办法是在聚合大括号内指定 size:
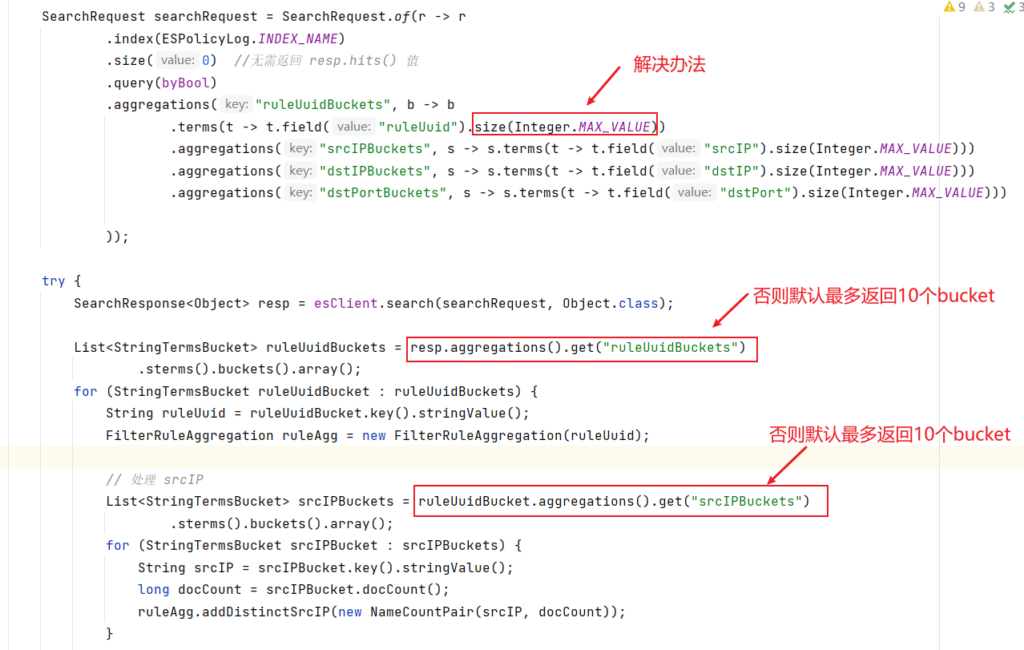